A multi-university research team co-led by University of Virginia engineering professor Gustavo K. Rohde has developed a system that can spot genetic markers of autism in brain images with 89 to 95% accuracy.
Their findings suggest doctors may one day see, classify and treat autism and related neurological conditions with this method, without having to rely on, or wait for, behavioral cues. And that means this truly personalized medicine could result in earlier interventions.
“Autism is traditionally diagnosed behaviorally but has a strong genetic basis. A genetics-first approach could transform understanding and treatment of autism,” the researchers wrote in a paper published June 12 in the journal Science Advances.
Rohde, a professor of biomedical and electrical and computer engineering, collaborated with researchers from the University of California San Franscisco and the Johns Hopkins University School of Medicine, including Shinjini Kundu, Rohde’s former Ph.D. student and first author of the paper.
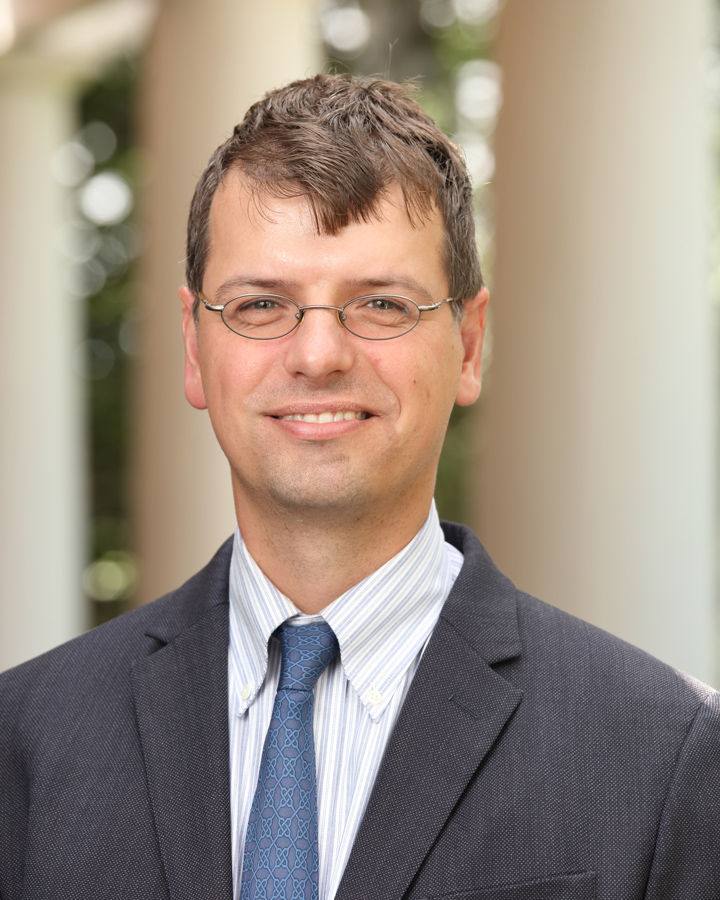
While working in Rohde’s lab, Kundu — now a physician at the Johns Hopkins Hospital — helped develop a generative computer modeling technique called transport-based morphometry, or TBM, which is at the heart of the team’s approach.
Using a novel mathematical modeling technique, their system reveals brain structure patterns that predict variations in certain regions of the individual’s genetic code — a phenomenon called “copy number variations,” in which segments of the code are deleted or duplicated. These variations are linked to autism.
TBM allows the researchers to distinguish normal biological variations in brain structure from those associated with the deletions or duplications.
“Some copy number variations are known to be associated with autism, but their link to brain morphology — in other words, how different types of brain tissues such as gray or white matter, are arranged in our brain — is not well known,” Rohde said. “Finding out how CNV relates to brain tissue morphology is an important first step in understanding autism’s biological basis.”
How TBM Cracks the Code
Transport-based morphometry is different from other machine learning image analysis models because the mathematical models are based on mass transport — the movement of molecules such as proteins, nutrients and gases in and out of cells and tissues. “Morphometry” refers to measuring and quantifying the biological forms created by these processes.
Most machine learning methods, Rohde said, have little or no relation to the biophysical processes that generated the data. They rely instead on recognizing patterns to identify anomalies.
But Rohde’s approach uses mathematical equations to extract the mass transport information from medical images, creating new images for visualization and further analysis.
Then, using a different set of mathematical methods, the system parses information associated with autism-linked CNV variations from other “normal” genetic variations that do not lead to disease or neurological disorders — what the researchers call “confounding sources of variability.”
Major discoveries from such vast amounts of data may lie ahead if we utilize more appropriate mathematical models to extract such information.
These sources previously prevented researchers from understanding the “gene-brain-behavior” relationship, effectively limiting care providers to behavior-based diagnoses and treatments.
According to Forbes magazine, 90% of medical data is in the form of imaging, which we don’t have the means to unlock. Rohde believes TBM is the skeleton key.
“As such, major discoveries from such vast amounts of data may lie ahead if we utilize more appropriate mathematical models to extract such information.”
The researchers used data from participants in the Simons Variation in Individuals Project, a group of subjects with the autism-linked genetic variation.
Control-set subjects were recruited from other clinical settings and matched for age, sex, handedness and non-verbal IQ while excluding those with related neurological disorders or family histories.
“We hope that the findings, the ability to identify localized changes in brain morphology linked to copy number variations, could point to brain regions and eventually mechanisms that can be leveraged for therapies,” Rohde said.
Publication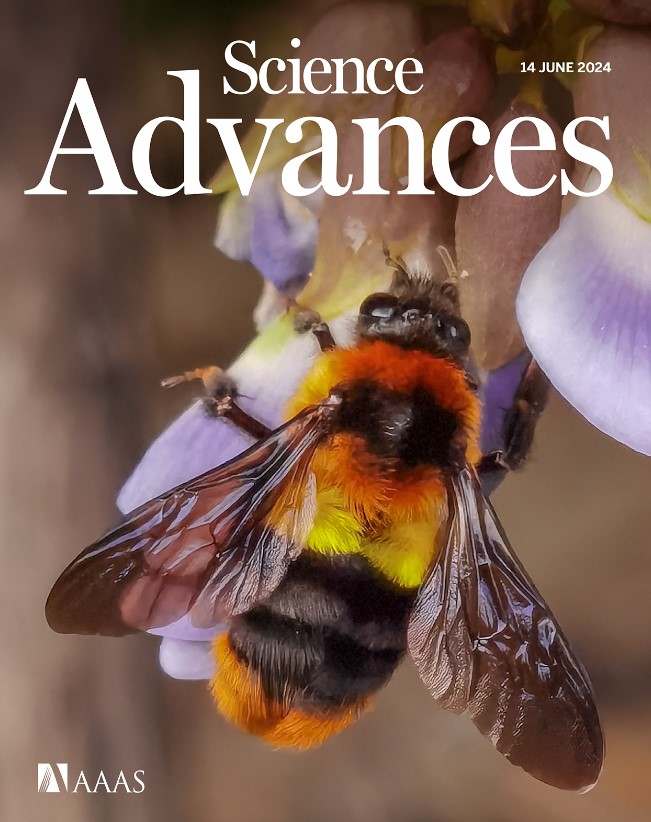
Discovering the gene-brain-behavior link in autism via generative machine learning was published online June 12, 2024, in Science Advances for the June 14 edition.
Additional co-authors are Haris Sair of the Johns Hopkins School of Medicine and Elliott H. Sherr and Pratik Mukherjee of the University of California San Francisco’s Department of Radiology.
The research received funding from the National Science Foundation, National Institutes of Health, Radiological Society of North America and the Simons Variation in Individuals Foundation.
This article was originally published by a engineering.virginia.edu . Read the Original article here. .